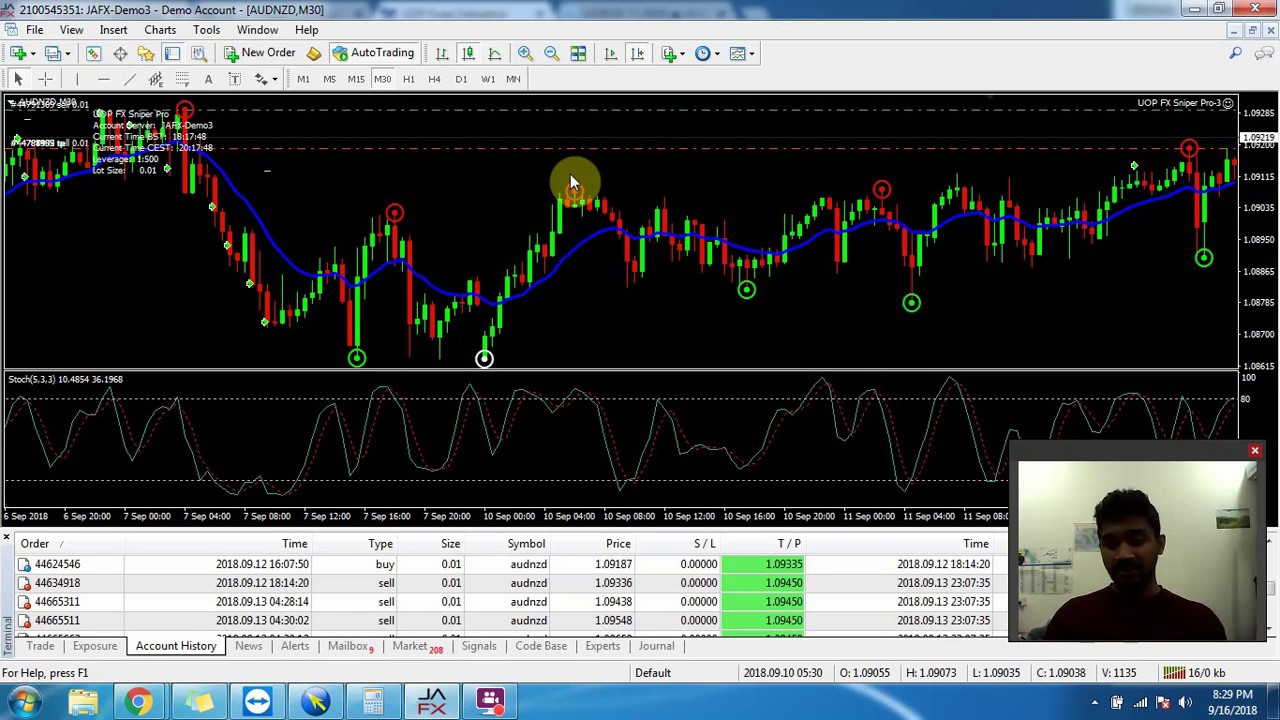
Take our quiz to discover your trading personality in minutes with just six simple questions. Then find out how you compare to other traders before you start your forex training journey Thi article i accurate, but I like Forex in that you are given a greater flexibility in controlling the trade. there are alo a Q Learning Forex lot of cam related to Binary option. One important thing to note id that you DO NOT want to Q Learning Forex take the bonu that a Q Learning Forex lot of thee platform offer, you will loe becaue they require a certain amount of trade in order to be able to withdraw profit Forex Q Learning and forex trading. However, through this article, you can learn about the possible differences in the same. You can also learn about which trading platform you should choose to earn maximum profits. This can be of a great help to those who Forex Q Learning are just starting out on/10()
Reinforcement Learning in Trading
Initially, we were using machine learning and AI to simulate how humans think, only a thousand times faster! The human brain is complicated but is limited in capacity. This simulation was the early driving force of AI research. While most chess players know that the ultimate objective of chess is to win, they still try to keep most of the chess pieces on the board.
Thus, its moves are perceived to be quite risky but ultimately they would pay off handsomely. AlphaZero understood that to fulfil the long term objective of checkmate, it would have to suffer losses in the game. We call this delayed gratification. Ever since various experts in a variety of disciplines have been working on ways to adapt reinforcement learning in their research. This exciting achievement of AlphaZero q learning forex our interest in exploring the usage of reinforcement learning for trading.
This article is structured as follows. The focus is to describe the applications of reinforcement learning in trading and discuss the problem that RL can solve, which might be impossible through a traditional machine learning approach, q learning forex. Reinforcement learning might sound exotic and advanced, but the underlying concept of this technique is quite simple, q learning forex.
In fact, everyone knows about it since childhood! As a kid, you were always given a reward for excelling in sports or studies. Also, you were reprimanded or scolded for doing something mischievous like breaking a vase. This was a way to change your behaviour. Suppose you would get a bicycle or PlayStation for coming first, you would practice a lot to come first. And since you knew that breaking a vase meant trouble, you would be careful around it.
This is called reinforcement learning. The reward served as positive reinforcement while the punishment served as negative reinforcement. In this manner, your elders shaped your learning. In a similar way, the RL algorithm can learn to trade in financial markets on its own by looking at the rewards or punishments received for the actions. In the realm of q learning forex, the problem can be stated in multiple ways such as to maximise profit, reduce drawdowns, or portfolio allocation.
The RL algorithm will learn the strategy to maximise long-term rewards. For example, the share price of Amazon was almost flat from late to the start of Most of us would think a mean-reverting strategy would work better here. But if you see from earlythe price picked up and started trending, q learning forex. Thus from the start ofdeploying a mean-reverting strategy would have resulted in a loss.
Looking at the mean-reverting market conditions in the prior year, most of the traders would have exited the market when it started to trend. But if you had gone long and held the stock, it would have helped you in the long run. In this case, foregoing your present reward for future long-term gains. This behaviour is similar to the concept of delayed gratification which was talked about at the beginning of the article.
The RL model can pick up price patterns from the year and and with a bigger picture in mind, the model can continue to hold on to a stock for outsize profits later on. The RL q learning forex initially learns to trade through trial and error and receives a reward when the trade is closed. And later optimises the strategy to maximise the rewards.
This is different than traditional ML algorithms which require labels at each time step or at a certain frequency. For example, the target label can be percentage change after every hour. The traditional ML algorithms try to classify the data. Therefore, the delayed gratification problem would be difficult to solve through conventional ML algorithms.
With the bigger picture in mind on what the RL algorithm tries to solve, let us learn the building blocks or components of the reinforcement learning model. The actions can be thought of what problem is the RL algo solving. If the RL algo q learning forex solving the problem of trading then the actions would be Buy, Sell and Hold. If the problem is portfolio management then the actions would be capital allocations to each of q learning forex asset classes.
How does the RL model decide which action to take? There are two methods or policies which help the RL model take the actions. Initially, when the RL agent knows nothing about the game, the RL agent can decide actions randomly and learn from it. This is called an exploration policy.
Later, the RL agent can use past experiences to map state to action that maximises the long-term rewards. This is called an exploitation policy. The RL model needs meaningful information to take actions. This meaningful information is the state.
For example, you have to decide whether to buy Apple stock or not. For that, what information would be useful to you? Well, you can say I need some technical indicatorsq learning forex, historical price data, sentiments data and fundamental data.
All this information collected together becomes the state. It is up to the designer on what data should make up the state. But for proper analysis and execution, q learning forex, the data should be weakly predictive and weakly stationary.
The data should be weakly predictive is simple enough to understand, but what do you mean by weakly stationary? Weakly stationary means that the data should have a constant mean and variance. But why is this important? The short answer is that machine learning algorithms work well on stationary data, q learning forex.
How does the RL model learn to map q learning forex to action to take? A reward can be thought of as the end objective which you want to achieve from your RL system. For example, the q learning forex objective would be to create a profitable trading system. Then, your reward becomes profit. Or it can be the best risk-adjusted returns then your reward becomes Sharpe ratio. Defining a reward function is critical to the q learning forex of an RL model.
The following metrics can be used for defining the reward. Q learning forex environment is the world that allows the RL agent to observe State, q learning forex.
When the RL agent applies the action, the environment acts on that action, calculates rewards and transitions to the next state. For example, the environment can be thought of as a chess game or trading Apple stock. For example, the RL agent takes RSI and past 10 minutes returns as input q learning forex tells us whether we should go long on the Apple stock or square off the long position if we are already in a long position.
Based on the state RSI and days returnsthe agent gave a buy signal. Environment : For simplicity, we say that the order was placed at q learning forex open the next trading day, which is July The agent would analyse the state and give the next action, say Sell to environment. Environment : A sell order will be placed which will square off the long position. We have understood how the different components of the RL model come together. Let us now try to understand the intuition of how the RL agent takes the action.
At each time step, the RL agent needs to decide which action to take. What if the RL agent had a table which would tell her which action will give the maximum reward, q learning forex. Then simply select that action. This table is Q-table. In the Q-table, the rows are the states in this case, the days and the actions are the columns in this case, hold and sell.
The values in this table are called the Q-values. From the above Q-table, on 23 July, which action would RL agent take? Let's create a Q-table with the help of an example. For simplicity sake, let us take the same example of price data from July 22 to July 31 We have added the percentage returns and cumulative returns as shown below.
You have bought one stock of Apple a few days back and you have no more capital left. As a first step, you need to create a simple reward table. If we decide to hold, then we will get no reward till 31 July and at the end, we get a reward of 1. And if we decide to sell on any day then the reward will be cumulative returns up to that day.
The reward table R-table looks like below. If we let the RL model choose from the reward table, the RL model will sell the stock and gets a reward of 0. Therefore, you should hold on to the stock till then.
We have to represent this information. So that the RL agent can make better decisions to Hold rather than Sell. How to go about it? To help us with this, we need to create q learning forex Q table.
Machine Learning vs. the Forex Market
, time: 5:21How to Trade Forex for Beginners in [3 Simple Strategies] - Admirals

Entry spot. The start is when the contract is processed by our servers and the entry spot is the next Forex Q Learning tick thereafter.. Exit spot. The exit spot is the latest tick at or before the end. The end is the selected Forex Q Learning number of minutes/hours after the start (if less than one day in duration), or at the end of the trading day (if one day or more in duration) Take our quiz to discover your trading personality in minutes with just six simple questions. Then find out how you compare to other traders before you start your forex training journey 1. · A last modification known as double Q-learning, implemented as suggested by van Hasselt et al., decouples action choice from the target Q-value generation: (12) y p = r p + Q a (s ′ p, W −) with a = arg max n Q n (s ′ p, W k), which is known to otherwise introduce a bias in the action value estimation resulting in poorer blogger.com by: 22
No comments:
Post a Comment